
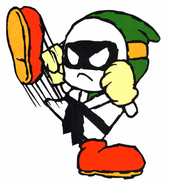
His research includes both theoretical and applied approaches to principal-agent problems, dynamic games, and information design. He received his PhD of Economics at Yale University, with a focus on information economics. It could be stolen but there is absolutely no dirt. Yi Chen is an Assistant Professor of Economics at Cornell University.

games of the General Video Game AI (GVGAI) framework. No commercial reproduction, distribution, display or performance rights in this work are provided. Linear Bandits with Limited Adaptivity and Learning Distributional Optimal. The bandits that raid Yi before Kai and Opal show up are driving a green jeep that Kuviras forces use. general approach that employs the N-Tuple Bandit Evolution. Yingyao Hu, Yutaka Kayaba, Matthew Shum, Nonparametric learning rules from bandit experiments: The eyes have it!, Games and Economic Behavior, Volume 81, September 2013, Pages 215-231, ISSN 0899-8256. Learning Belief dynamics Experiments Eye tracking Bayesian vs. Kayaba thanks the Nakajima Foundation for the financial support. Washington and Choice Symposium 2010 (Key Largo) for comments and suggestions. Power up to faster cars, get the edge with getaway gadgets and outrun the cops as you speed to the state line. From the makers of Smash Cops crash through America in the craziest road race to hit the Google Play Store. We thank Dan Ackerberg, Peter Bossaerts, Colin Camerer, Andrew Ching, Mark Dean, Cary Frydman, Ian Krajbich, Pietro Ortoleva, Joseph Tao-yi Wang and participants in presentations at U. by Chen, Yi-Chun & Kunimoto, Takashi & Sun, Yifei & Xiong, Siyang. Named as one of TouchArcade's Top 10 Games of the year. We are indebted to Antonio Rangel for his encouragement and for the funding and use of facilities in his lab. Received 22 February 2012 Available online. The profits from following the estimated learning and decision rules are smaller (by about 25% of average earnings by subjects in this experiment) than what would be obtained from a fully-rational Bayesian learning model, but comparable to the profits from alternative non-Bayesian learning models, including reinforcement learning and a simple “win-stay” choice heuristic. Estimates show that subjects are more reluctant to “update down” following unsuccessful choices, than “update up” following successful choices. Instead of estimating the cumulative distribution, here we view the. A novel feature of our approach is to supplement the choice and reward data with subjectsʼ eye movements during the experiment to pin down estimates of subjectsʼ beliefs. Lei Xu, Chunxiao Jiang, Yi Qian, Yong Ren. How do people learn? We assess, in a model-free manner, subjectsʼ belief dynamics in a two-armed bandit learning experiment.
